Making Better Beer and Wine with Data and Machine Learning
GPS-Wearing Dogs, an Electronic Nose, and a Robot That Pours the Perfect Beer
Bushfires in Australia are as commonplace as kangaroos and koalas. A hot, dry climate regularly sets the stage for conflagrations that endanger human lives, property, and wildlife and threaten one of the country’s top economic industries: wine. Fires during summer 2019–2020 decimated entire vineyards in South Australia, Victoria and New South Wales, but smoke, which was far more widespread and insidious, seeped into grapes and into fermenting barrels, yielding unpleasant, unsaleable product. Although the full extent of the damage caused has not yet been calculated, analysis from the Australian Wine Research Institute indicates that smoke taint alone costs the country’s wine industry tens to hundreds of millions of dollars each time a high fire season occurs.
“This research could take out the guesswork from viticulture and winemaking, making them more predictable”
Advances in a wide range of technologies could help growers and winemakers mitigate the negative impact of smoke taint and other unpredictable anomalies, such as frost, drought, pests, and disease — and not just in Australia, but around the world. The Vineyard of the Future, led by Associate Professor Sigfredo Fuentes, a plant physiologist at the University of Melbourne, is an international consortium of scientists conducting leading-edge research to amass high-resolution data from vine to glass and analyze it in meaningful ways. Drones, satellite imaging, video analysis, and plant and people sensors combined with artificial intelligence—collectively called “digital agriculture”—give producers and sellers of wine an advantage in an industry riddled with uncertainty.
“This research could take out the guesswork from viticulture and winemaking, making them more predictable,” says Fuentes.
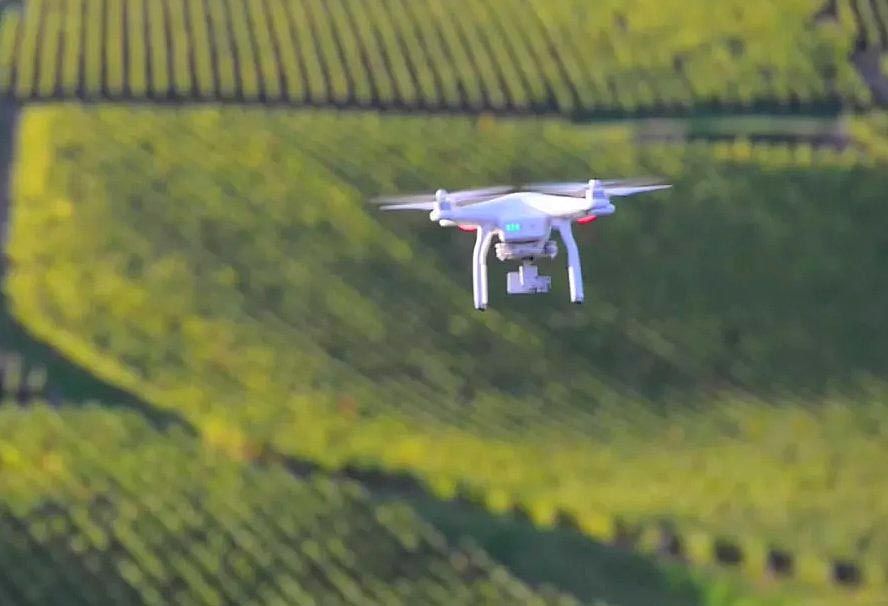
On the Vine
Good wine starts on the vine. Delectable grapes depend on the weather and cultivation strategies, including irrigation, fertilization, pest control, and canopy management. Growers typically prefer smaller grape berries, which yield more grape skin and therefore more compounds, such as anthocyanins, tannins, resveratrol, and polyphenols, that influence flavor and aroma. Lower yields of grapes with top-quality traits may actually produce higher revenue per acre, so it’s essential to maintain the balance between the vegetative and reproductive sections of grapevines, says Fuentes. “No recipe fits all cases for viticulture, and here the implementation of new and emerging technologies is critical to assess all these factors to obtain good products,” he says.
Fuentes and his colleagues have developed technologies that rely on infrared thermal imagery and near infrared spectroscopy (NIR) analysis coupled with supervised machine learning modeling to measure smoke contamination in leaves and assess smoke taint in the grapes. Infrared cameras reveal a vine’s heat signature, which is disrupted by smoke. Using MATLAB®, Fuentes and his team developed computer vision algorithms that use the heat signature to predict smoke contamination in canopies with 96% accuracy.
NIR data obtained using non-invasive handheld instruments reveals a chemical fingerprint from berries and wines that indicates specific smoke-related compounds and concentrations in near real time with high accuracy. Conventional methods available to growers require them to send grapes to a lab and wait six days or more for the results. But having the information in real time could help growers make decisions, such as whether to harvest untainted grapes separate from tainted ones, in order to minimize waste.
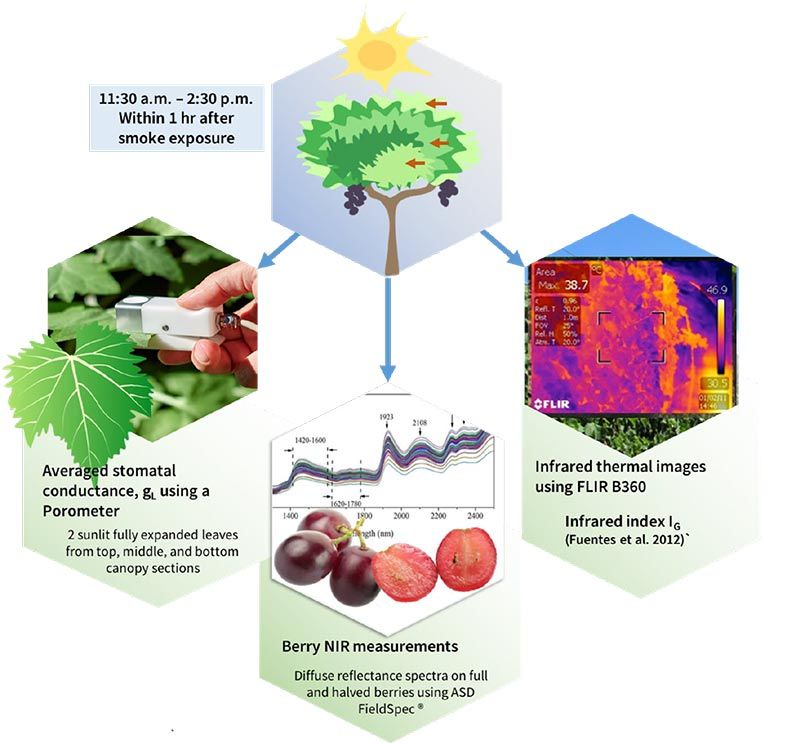
Machine learning detects grape contamination from bush fires within one hour after exposure. Image credit: Dr. Eden Tongson
Fuentes and his team developed computer vision algorithms that use a vine’s heat signature to predict smoke contamination with 96% accuracy.
Further research has been conducted into predicting quality traits of potential wines from vineyards even before harvest. By incorporating other variables such as weather data inputs and known aroma profiles from previous vintages as targets, machine learning models were trained to predict the aroma profile of the wine coming from the vines.
An app called VitiCanopy uses a smartphone’s GPS and camera to help a grower measure a canopy’s size, density, and vigor. From an image, the app’s computer vision algorithm calculates the leaf area index with a snapshot. Known as LAI, this important metric correlates the amount of sunlight dappling the berries, the canopy’s microclimate, the grape’s composition, and ultimately yield. Wine growers are trying to create a balance between the leaves, the shoots, and the fruit, says Fuentes. “If you have too vigorous of a canopy, the flavor and aroma profiles of the final wine are going to have too much acidity and green respectively,” he says. The information from the app enables a grower to make decisions about trimming a canopy, applying fertilizer, and increasing or decreasing irrigation. “It's all about balance,” says Fuentes.
NIR and machine learning algorithms can also lend clues to grape ripeness. Fuentes explains that certain compounds, released from dying cells inside the grape as it ripens, influence its aroma and flavor. Different grapes require different percentages of cellular death to reach their peak ripeness. “We propose measuring the cell vitality of berries before doing the winemaking to predict the quality of the wine using digital tools developed,” he says.
On the Nose
Among the dozens of variables affecting—and potentially devastating—vineyards, an insect called phylloxera may be one of the most notorious. In the mid-19th century, French vintners unknowingly imported the insect from the United States when they brought American vine material, contaminated shoes or tools to Europe. Although phylloxera preferred to dine harmlessly on the leaves of American vines, when they discovered French vines, they went for the roots. The Great French Wine Blight nearly decimated the country’s wine industry in just a couple of decades. After several failed attempts to eradicate the insect, French vintners begrudgingly grafted their vines to American vine roots, creating plants that could thrive in French soils and resist phylloxera.
Today, phylloxera remains a threat that is difficult to detect. Early signs, such as leaf discoloration and general canopy wilting, are frequently confused for water and fertilizer stress, and vintners are motivated to find a more reliable identification method. One approach has gone to the dogs—in a good way. With a nose containing 300 million more smell receptors than a human’s, resulting in 100 times the sensitivity, dogs are being trained by researchers in the Vineyard of the Future to recognize the scent of pheromones released by phylloxera insects as well as other chemical compounds produced by the insect.
A low-cost, portable electronic nose with an array of sensors that can detect nine different gases could be tweaked to identify smoke damage in vineyards.
Wearing a backpack equipped with GPS-enabled smartphone, a dog will traverse a vineyard, its nose to the ground. Tracking algorithms developed using MATLAB Mobile™ detect the dog’s location as well as its motion. Different actions such as running, walking, and sitting when a scent is detected are added to a map to pinpoint problems in vineyard. “The app creates a log file for all of the points where the dog signals the handler by sitting, crouching or scratching,” says Claudia Gonzalez Viejo, a postdoctoral fellow working with Fuentes. The grower can then target inspections to those locations, saving time.
While the dogs were highly skilled at locating pests, they weren’t very good at determining the perfect beer aroma. To supplement the expert dog noses, Fuentes and his team have developed a low-cost, portable electronic nose, or e-nose, that has an array of sensors that are able to detect nine different gases, including ethanol, carbon dioxide, carbon oxide, methane, and hydrogen peroxide. Gonzalez Viejo helped design the e-nose to scrutinize beer samples and predict aromas. But, she says, it has a wide range of applications, and could be tweaked to detect smoke damage in vineyards. Gonzalez Viejo envisions the technology being combined with a dog detector, used as a handheld device, or mounted to a drone and flown down rows. “We can take the e-nose anywhere,” she says.
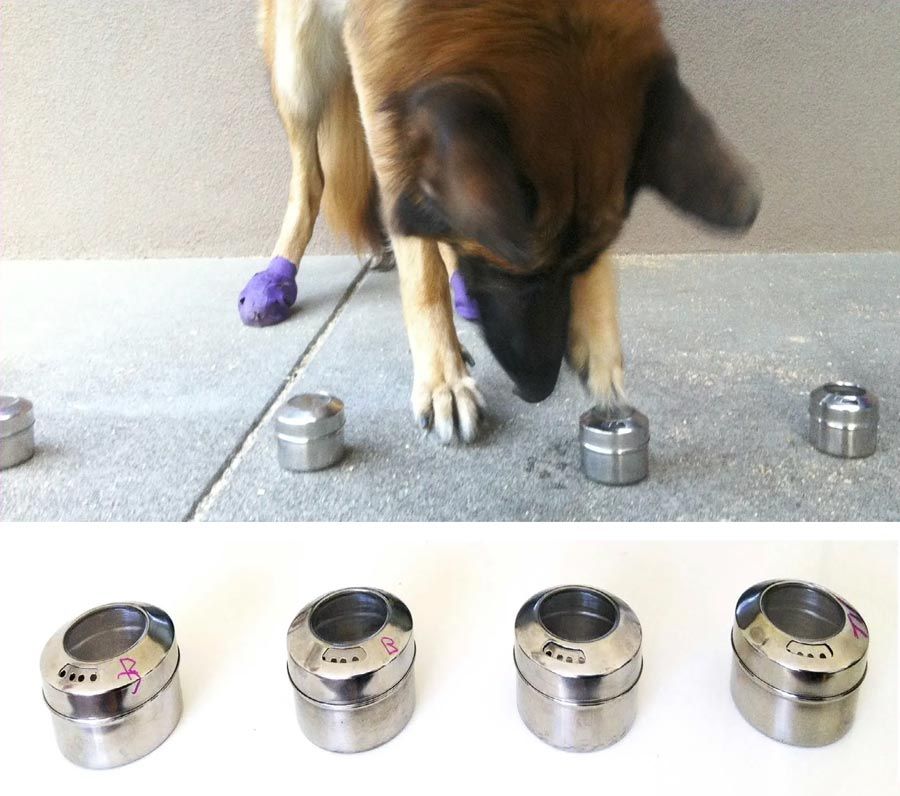
A specially trained dog moves along a line of containers and correctly indicates with a focused stare and touch of the paw. Image credit: Sonja Needs
In the Glass
For all of the work growers and producers do to make a quality wine, what constitutes good is subjective. “The best wine is the wine that you like,” says Fuentes.
Ultimately, understanding consumer reaction is key to selling wine, and Vineyard of the Future researchers have developed technology for that, too. The system, which was refined using beer but applies to wine and sparkling wine, incorporates a robotic arm, cameras, and the e-nose. It begins with a perfect pour from the robotic arm, designed to fill a glass the same way each time without tiring. High-definition cameras trained on the beer capture visual data, including color, foam formation and dissipation, and bubble size. An e-nose, positioned over the top of the glass, measures the gases released. Computer vision and machine learning algorithms crunch the camera and sensor information and position the beer amid a library of 250 others that have been previously analyzed.
Aroma profiles conducted with the sensors were 97% accurate. Such technology could be attractive to craft brewers interested in maintaining consistency and quality control. “You can test every batch and get instant results,” says Gonzalez Viejo.
The team has even added consumer reaction to mix. Researchers used video cameras, infrared thermal imagery, and brain wave headsets to measure heart rate, body temperature, brainwaves, and facial expressions of staff and student participants as they drank different beers and wines or consumed food products. Their assessments of foam, color, aroma, mouthfeel, taste, flavor, and overall likeness of beers are being paired with the visual and e-nose data collected during a pour to increase accuracy.
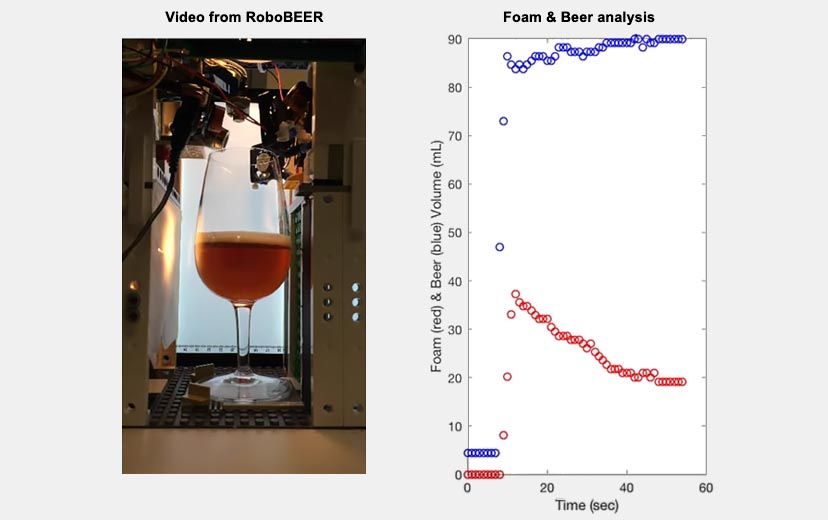
RoboBEER uses computer vision algorithms, artificial neural networks, and visual characteristics such as foam, color, and bubbles to create the perfect pour every time. Image credit: The University of Melbourne
Viticulture, winemaking, and brewing are part art form, part science. As technology advances and scientific understanding of the processes occurring in the soil, the root system, the plant, the canopies, and the atmosphere deepens, science may find an advantage. As wine and beer become more popular and demand grows, especially in a world with changing climate, emerging technologies could give growers and producers something to toast.