メインコンテンツ
結果:
Curve fitting is a fundamental step in modeling and prediction during data analysis. This script demonstrates how to create an interactive fitting tool in MATLAB that allows users to perform first- or second-order polynomial or exponential fitting on two different datasets. It also compares the fitting results between the original data and scaled data (normalized by subtracting the minimum value).
clc;
clear;
% Basic data definition
x = [1,2,3,5,15,25,40,60]';
y1 = [0.5,0.3,0.8,1,1.5,0.5,1,2]';
y2 = [45,46,44.5,45,44.8,45.5,45,48]';
% Create denser x values for smooth curves
x_dense = linspace(min(x), max(x), 1000)'; % 1000 points
% User input
disp('Please choose the dataset to analyze:');
disp('1 = Dataset 1 (y1)');
disp('2 = Dataset 2 (y2)');
dataset = input('Enter your choice (1 or 2): ');
disp('Please choose the fitting type:');
disp('1 = Polynomial fitting');
disp('2 = Exponential fitting');
fit_type = input('Enter your choice (1 or 2): ');
if fit_type == 1
disp('Please choose the polynomial order:');
order = input('Enter order (1 or 2): ');
else
disp('Please choose the exponential order:');
order = input('Enter order (1 or 2): ');
end
% Select dataset
if dataset == 1
y = y1;
else
y = y2;
end
% Create figure window
figure;
% Plot original data
subplot(1,2,1);
plot(x, y, 'bo');
hold on;
title('Original Data Fitting');
xlabel('x');
ylabel('y');
% Plot scaled data
y_scaled = y - min(y);
subplot(1,2,2);
plot(x, y_scaled, 'ro');
hold on;
title('Scaled Data Fitting');
xlabel('x');
ylabel('y\_scaled');
% Perform fitting
switch fit_type
case 1 % Polynomial fitting
switch order
case 1
p = polyfit(x, y, 1);
p_scaled = polyfit(x, y_scaled, 1);
% Calculate fitted values at dense points
y_fit = polyval(p, x_dense);
y_fit_scaled = polyval(p_scaled, x_dense);
fit_name = '1st Order Polynomial';
case 2
p = polyfit(x, y, 2);
p_scaled = polyfit(x, y_scaled, 2);
y_fit = polyval(p, x_dense);
y_fit_scaled = polyval(p_scaled, x_dense);
fit_name = '2nd Order Polynomial';
end
case 2 % Exponential fitting
switch order
case 1
f = fit(x, y, 'exp1');
fs = fit(x, y_scaled, 'exp1');
% Calculate fitted values at dense points
y_fit = f.a * exp(f.b * x_dense);
y_fit_scaled = fs.a * exp(fs.b * x_dense);
fit_name = '1st Order Exponential';
case 2
f = fit(x, y, 'exp2');
fs = fit(x, y_scaled, 'exp2');
y_fit = f.a * exp(f.b * x_dense) + f.c * exp(f.d * x_dense);
y_fit_scaled = fs.a * exp(fs.b * x_dense) + fs.c * exp(fs.d * x_dense);
fit_name = '2nd Order Exponential';
end
end
% Plot smooth fitted curves
subplot(1,2,1);
plot(x_dense, y_fit, 'r-', 'LineWidth', 1.5);
legend('Original Data', [fit_name ' Fit']);
subplot(1,2,2);
plot(x_dense, y_fit_scaled, 'r-', 'LineWidth', 1.5);
legend('Scaled Data', [fit_name ' Fit']);
% Compute fitting metrics (still based on original data points)
switch fit_type
case 1
y_fit_original = polyval(p, x);
y_fit_scaled_original = polyval(p_scaled, x);
case 2
y_fit_original = f.a * exp(f.b * x) + f.c * exp(f.d * x);
y_fit_scaled_original = fs.a * exp(fs.b * x) + fs.c * exp(fs.d * x);
end
SSE = sum((y - y_fit_original).^2);
SST = sum((y - mean(y)).^2);
R2 = 1 - SSE/SST;
SSE_scaled = sum((y_scaled - y_fit_scaled_original).^2);
SST_scaled = sum((y_scaled - mean(y_scaled)).^2);
R2_scaled = 1 - SSE_scaled/SST_scaled;
% Display results
fprintf('\n%s Fitting Results:\n', fit_name);
fprintf('Original Data - Sum of Squared Errors: %.4f, R-squared: %.4f\n', SSE, R2);
fprintf('Scaled Data - Sum of Squared Errors: %.4f, R-squared: %.4f\n', SSE_scaled, R2_scaled);
I'm getting this annoying survey (screenshot below) in the help windows of MATLAB R2024b this morning. It blocks the text I'm actually trying to read, when minimised it pops up again after a few minutes, and persists even after picking an option and completing the SurveyMonkey survey it links to. I don't even know what the OPC UA server so rest assured any of my answers to that survey aren't going to help MathWorks improve their product.
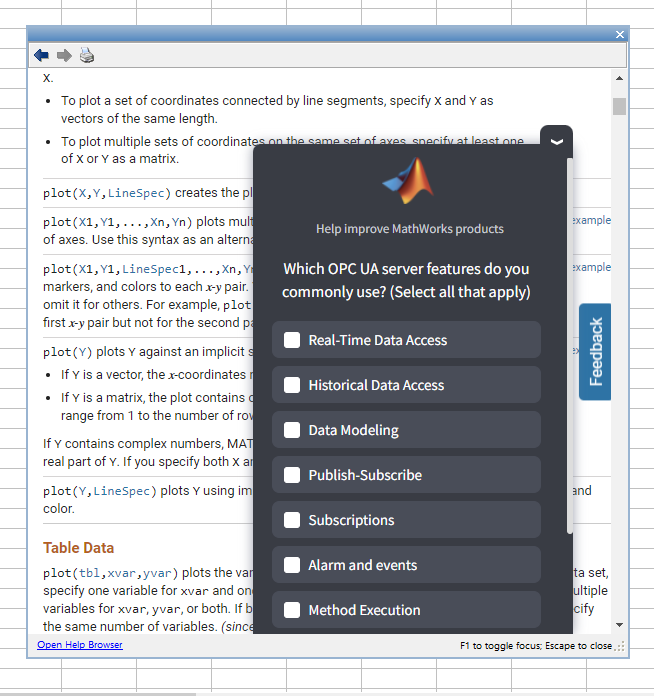
I've long used the Tensor Toolbox from Sandia in order to use tensors in Matlab, but recently found myself wanting to apply it on symbolic arguments, which don't appear supported. Some google-fu'ing resulted in (non-free) Tensorlab and some file-exchange entries of mixed quality. And of course, there's the recent tensorprod, which a) doesn't support symbolics and b) arguments aren't strictly tensors (rather "representations of tensors in a matrix type").
This all got me to thinking that it would be mighty nice to have general / native / comprehensive support for a tensor class in official Matlab - even if it were in a separate toolbox.
It is April 3, 2025 now. Where is the MATLAB 2025a?
Me: If you have parallel code and you apply this trick that only requires changing one line then it might go faster.
Reddit user: I did and it made my code 3x faster
Not bad for just one line of code!
Which makes me wonder. Could it make your MATLAB program go faster too? If you have some MATLAB code that makes use of parallel constructs like parfor or parfeval then start up your parallel pool like this
parpool("Threads")
before running your program.
The worst that will happen is you get an error message and you'll send us a bug report....or maybe it doesn't speed up much at all....
....or maybe you'll be like the Reddit user and get 3x speed-up for 10 seconds work. It must be worth a try...after all, you're using parallel computing to make your code faster right? May as well go all the way.
In an artificial benchmark I tried, I got 10x speedup! More details in my recent blog post: Parallel computing in MATLAB: Have you tried ThreadPools yet? » The MATLAB Blog - MATLAB & Simulink
Give it a try and let me know how you get on.
Hello Community,
We're excited to announce that registration is now open for the MathWorks AUTOMOTIVE CONFERENCE 2025! This event presents a fantastic opportunity to connect with MathWorks and industry experts while exploring the latest trends in the automotive sector.
Event Details:
- Date: April 29, 2025
- Location: St. John’s Resort, Plymouth, MI
Featured Topics:
- Virtual Development
- Electrification
- Software Development
- AI in Engineering
Whether you're a professional in the automotive industry or simply interested in these cutting-edge topics, we highly encourage you to register for this conference.
We look forward to seeing you there!
We are excited to announce another update to our Discussions area: the new Contribution Widget! The new widget simplifies the process of creating diverse types of content, whether you're praising someone who has helped you, sharing tips and tricks, or polling the community.
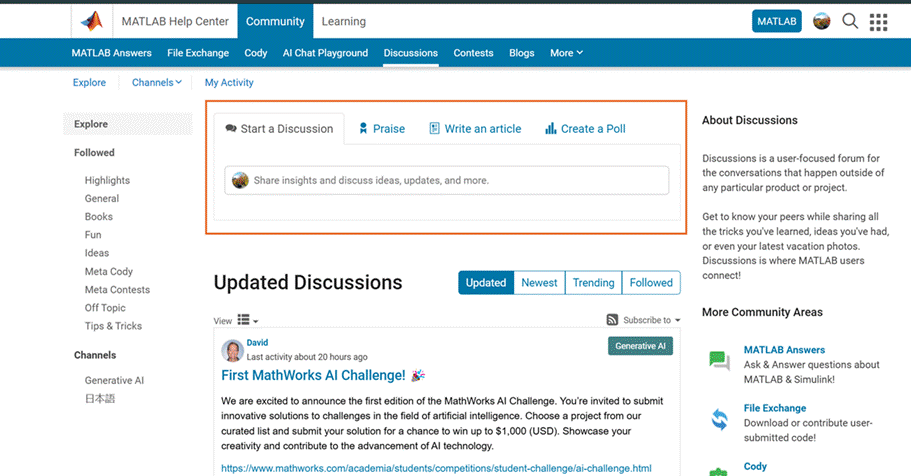
Previously, creating various types of content required navigating multiple links or channels. With the new Contribution Widget, everything you need is conveniently located in one place.
Give it a try and let us know how we can further enhance your user experience.
P.S. Who has been particularly helpful to you lately? Create your first praise post and let them know!
I am pleased to announce the 6th Edition of my book MATLAB Recipes for Earth Sciences with Springer Nature
also in the MathWorks Book Program
It is now almost exactly 20 years since I signed the contract with Springer for the first edition of the book. Since then, the book has grown from 237 to 576 pages, with many new chapters added. I would like to thank my colleagues Norbert Marwan and Robin Gebbers, who have each contributed two sections to Chapters 5, 7 and 9.
And of course, my thanks go to the excellent team at the MathWorks Book Program and the numerous other MathWorks experts who have helped and advised me during the last 30+ years working with MATLAB. And of course, thank you Springer for 20 years of support.
This book introduces methods of data analysis in the earth sciences using MATLAB, such as basic statistics for univariate, bivariate, and multivariate data sets, time series analysis, signal processing, spatial and directional data analysis, and image analysis.
Martin H. Trauth
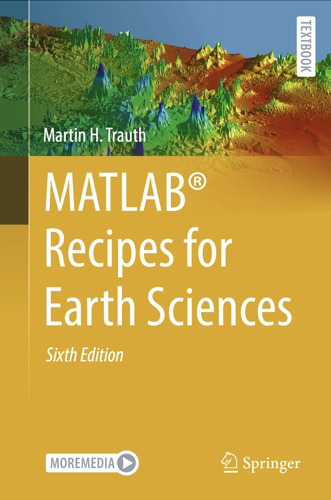
There has been a lot of discussion here about the R2025a Prerelease that has really helped us get it ready for the prime time. Thank you for that!
A new update of the Prerelease has just dropped. So fresh it is still warm from the oven! In my latest blog post I discuss changes in the way MathWorks has been asking-for and processing feedback...and you have all been a part of that.
If you haven't tried the Prerelease in a while, I suggest you update and see how things are looking now.
If you have already submitted a bug report and it hasn't been fixed in this update, you don't need to submit another one. Everything is being tracked!
Have a play, discuss it here and thanks for again for being part of the process.
📢 We want to hear from you! We're a team of graduate student researchers at the University of Michigan studying MATLAB Drive and other cloud-based systems for sharing coding files. Your feedback will help improve these tools. Take our quick survey here: https://forms.gle/DnHs4XNAwBZvmrAw6
No
50%
Yes, but I am not interested
7%
Yes, but it is too expensive
20%
Yes, I would like to know more
18%
Yes, I am cert. MATLAB Associate
2%
Yes, I am cert. MATLAB Professional
3%
4393 票
Imagine you are developing a new toolbox for MATLAB. You have a folder full of a few .m files defining a bunch of functions and you are thinking 'This would be useful for others, I'm going to make it available to the world'
What process would you go through? What's the first thing you'd do?
I have my own opinions but don't want to pollute the start of the conversation :)
I am glad to inform and share with you all my new text book titled "Inverters and AC Drives
Control, Modeling, and Simulation Using Simulink", Springer, 2024. This text book has nine chapters and three appendices. A separate "Instructor Manual" is rpovided with solutions to selected model projects. The salent features of this book are given below:
- Provides Simulink models for various PWM techniques used for inverters
- Presents vector and direct torque control of inverter-fed AC drives and fuzzy logic control of converter-fed AC drives
- Includes examples, case studies, source codes of models, and model projects from all the chapters
The Springer link for this text book is given below:
This book is also in the Mathworks book program:
Over the last 5 years or so, the highest-traffic post on my MATLAB Central image processing blog was not actually about image processing; it was about changing the default line thickness in plots.
Now I have written about some other MATLAB plotting behavior that I have recently changed to suit my own preferences. See this new blog post.
Here is a standard MATLAB plot:
x = 0:pi/100:2*pi;
y1 = sin(x);
y2 = cos(x);
plot(x,y1,x,y2)
I don't like some aspects of this plot, and so I have put the following code into my startup file.
set(groot,"DefaultLineLineWidth",2)
set(groot,"DefaultAxesXLimitMethod","padded")
set(groot,"DefaultAxesYLimitMethod","padded")
set(groot,"DefaultAxesZLimitMethod","padded")
set(groot,"DefaultAxesXGrid","on")
set(groot,"DefaultAxesYGrid","on")
set(groot,"DefaultAxesZGrid","on")
With those defaults changed, here is my preferred appearance:
plot(x,y1,x,y2)
To develop uifigure-based app, I wish MATLAB can provide something like uiquestdlg to replace questdlg without changing too much of the original code developed for figure-based app. Also, uiinputdlg <-> inputdlg and so on.
It is time to support the cameraIntrinsics function to accept a 3-by-3 intrinsic matrix K as an input parameter for constructing the object. Currently, the built-in cameraIntrinsics function can only be constructed by explicitly specifying focalLength, principalPoint, and imageSize. This approach has drawbacks, as it is not very intuitive. In most application scenarios, using the intrinsic matrix
K=[fx,0,cx;
0,fy,cy;
0,0,1]
is much more straightforward and effective!
intrinsics = cameraIntrinsics(K)
Learn the basic of quantum computing, how to simulate quantum circuits on MATLAB and how to run them on real quantum computers using Amazon Braket. There will also be a demonstration of machine learning using quantum computers!
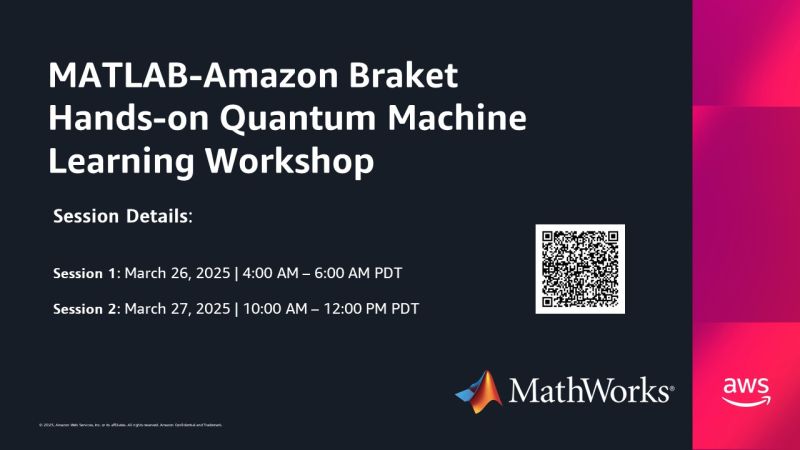
Details at MATLAB-AMAZON Braket Hands-on Quantum Machine Learning Workshop - MATLAB & Simulink. This will be led by MathWorker Hossein Jooya.
I kicked off my own exploration of Quantum Computing in MATLAB a year or so ago and wrote it up on The MATLAB Blog: Quantum computing in MATLAB R2023b: On the desktop and in the cloud » The MATLAB Blog - MATLAB & Simulink. This made use of the MATLAB Support Package for Quantum Computing - File Exchange - MATLAB Central
Good day I am looking someone to help me on the matlab and simulink I am missing some explanations.For easy communication you can contact 0026876637042
Recently my iMac became sluggish. I checked Activity Monitor and found it was spending most of its time in mds_stores. I turned of Apple Intelligence under System Settings - Apple Intelligence & Siri, and its like new again.
The GCD approach to identify rough numbers is a terribly useful one, well worth remembering. But at some point, I expect someone to notice that all work done with these massively large symbolic numbers uses only one of the cores on your computer. And, having spent so much money on those extra cores in your CPU, surely we can find a way to use them all? The problem is, computations done on symbolic integers never use more than 1 core. (Sad, unhappy face.)
In order to use all of the power available to your computer using MATLAB, you need to work in double precision, or perhaps int64 or uint64. To do that, I'll next search for primes among the family 3^n+4. In fact, they seem pretty common, at least if we look at the first few such examples.
F = @(n) sym(3).^n + 4;
F(0:16)
ans =
[5, 7, 13, 31, 85, 247, 733, 2191, 6565, 19687, 59053, 177151, 531445, 1594327, 4782973, 14348911, 43046725]
isprime(F(0:16))
ans =
1×17 logical array
1 1 1 1 0 0 1 0 0 1 1 0 0 0 0 0 0
Of the first 11 members of that sequence, 7 of them were prime. Naturally, primes will become less frequent in this sequence as we look further out. The members of this family grow rapidly in size. F(10000) has 4771 decimal digits, and F(100000) has 47712 decimal digits. We certainly don't want to directly test every member of that sequence for primality. However, what I will call a partial or incomplete sieve can greatly decrease the work needed.
Consider there are roughly 5.7 million primes less than 1e8.
numel(primes(1e8))
ans =
5761455
F(17) is the first member of our sequence that exceeds 1e8. So we can start there, since we already know the small-ish primes in this sequence.
roughlim = 1e8;
primes1e8 = primes(roughlim);
primes1e8([1 2]) = []; % F(n) is never divisible by 2 or 3
F_17 = double(F(17));
Fremainders = mod(F_17,primes1e8);
nmax = 100000;
FnIsRough = false(1,nmax);
for n = 17:nmax
if all(Fremainders)
FnIsRough(n) = true;
end
% update the remainders for the next term in the sequence
% This uses the recursion: F(n+1) = 3*F(n) - 8
Fremainders = mod(Fremainders*3 - 8,primes1e8);
end
sum(FnIsRough)
ans =
6876
These will be effectively trial divides, even though we use mod for the purpose. The result is 6876 1e8-rough numbers, far less than that total set of 99984 values for n. One thing of great importance is to recognize this sequence of tests will use an approximately constant time per test regardless of the size of the numbers because each test works off the remainders from the previous one. And that works as long as we can update those remainders in some simple, direct, and efficient fashion. All that matters is the size of the set of primes to test against. Remember, the beauty of this scheme is that while I did what are implicitly trial divides against 5.76 million primes at each step, ALL of the work was done in double precision. That means I used all 8 of the cores on my computer, pushing them as hard as I could. I never had to go into the realm of big integer arithmetic to identify the rough members in that sequence, and by staying in the realm of doubles, MATLAB will automatically use all the cores you have available.
The first 10 values of n (where n is at least 17), such that F(n) is 1e8-rough were
FnIsRough = find(FnIsRough);
FnIsRough(1:10)
ans =
22 30 42 57 87 94 166 174 195 198
How well does the roughness test do to eliminate composite members of this sequence?
isprime(F(FnIsRough(1:10)))
ans =
1×10 logical array
1 1 1 1 1 0 0 1 1 1
As you can see, 8 of those first few 1e8-rough members were actually prime, so only 2 of those eventual isprime tests were effectively wasted. That means the roughness test was quite useful indeed as an efficient but relatively weak pre-test for possible primality. More importantly it is a way to quickly eliminate those values which can be known to be composite.
You can apply a similar set of tests on many families of numbers. For example, repunit primes are a great case. A rep-digit number is any number composed of a sequence of only a single digit, like 11, 777, and 9999999999999.
However, you should understand that only rep-digit numbers composed of entirely ones can ever be prime. Naturally, any number composed entirely of the digit D, will always be divisible by the single digit number D, and so only rep-unit numbers can be prime. Repunit numbers are a subset of the rep-digit family, so numbers composed only of a string of ones. 11 is the first such repunit prime. We can write them in MATLAB as a simple expression:
RU = @(N) (sym(10).^N - 1)/9;
RU(N) is a number composed only of the digit 1, with N decimal digits. This family also follows a recurrence relation, and so we could use a similar scheme as was used to find rough members of the set 3^N-4.
RU(N+1) == 10*RU(N) + 1
However, repunit numbers are rarely prime. Looking out as far as 500 digit repunit numbers, we would see primes are pretty scarce in this specific family.
find(isprime(RU(1:500)))
ans =
2 19 23 317
There are of course good reasons why repunit numbers are rarely prime. One of them is they can only ever be prime when the number of digits is also prime. This is easy to show, as you can always factor any repunit number with a composite number of digits in a simple way:
1111 (4 digits) = 11*101
111111111 (9 digits) = 111*1001001
Finally, I'll mention that Mersenne primes are indeed another example of repunit primes, when expressed in base 2. A fun fact: a Mersenne number of the form 2^n-1, when n is prime, can only have prime factors of the form 1+2*k*n. Even the Mersenne number itself will be of the same general form. And remember that a Mersenne number M(n) can only ever be prime when n is itself prime. Try it! For example, 11 is prime.
Mn = @(n) sym(2).^n - 1;
Mn(11)
ans =
2047
Note that 2047 = 1 + 186*11. But M(11) is not itself prime.
factor(Mn(11))
ans =
[23, 89]
Looking carefully at both of those factors, we see that 23 == 1+2*11, and 89 = 1+8*11.
How does this help us? Perhaps you may see where this is going. The largest known Mersenne prime at this date is Mn(136279841). This is one seriously massive prime, containing 41,024,320 decimal digits. I have no plans to directly test numbers of that size for primality here, at least not with my current computing capacity. Regardless, even at that realm of immensity, we can still do something.
If the largest known Mersenne prime comes from n=136279841, then the next such prime must have a larger prime exponent. What are the next few primes that exceed 136279841?
np = NaN(1,11); np(1) = 136279841;
for i = 1:10
np(i+1) = nextprime(np(i)+1);
end
np(1) = [];
np
np =
Columns 1 through 8
136279879 136279901 136279919 136279933 136279967 136279981 136279987 136280003
Columns 9 through 10
136280009 136280051
The next 10 candidates for Mersenne primality lie in the set Mn(np), though it is unlikely that any of those Mersenne numbers will be prime. But ... is it possible that any of them may form the next Mersenne prime? At the very least, we can exclude a few of them.
for i = 1:10
2*find(powermod(sym(2),np(i),1+2*(1:50000)*np(i))==1)
end
ans =
18 40 64
ans =
1×0 empty double row vector
ans =
2
ans =
1×0 empty double row vector
ans =
1×0 empty double row vector
ans =
1×0 empty double row vector
ans =
1×0 empty double row vector
ans =
1×0 empty double row vector
ans =
1×0 empty double row vector
ans =
2
Even with this quick test which took only a few seconds to run on my computer, we see that 3 of those Mersenne numbers are clearly not prime. In fact, we already know three of the factors of M(136279879), as 1+[18,40,64]*136279879.
You might ask, when is the MOD style test, using a large scale test for roughness against many thousands or millions of small primes, when is it better than the use of GCD? The answer here is clear. Use the large scale mod test when you can easily move from one member of the family to the next, typically using a linear recurrence. Simple such examples of this are:
1. Repunit numbers
General form: R(n) = (10^n-1)/9
Recurrence: R(n+1) = 10*R(n) + 1, R(0) = 1, R(1) = 11
2. Fibonacci numbers.
Recurrence: F(n+1) = F(n) + F(n-1), F(0) = 0, F(1) = 1
3. Mersenne numbers.
General form: M(n) = 2^n - 1
Recurrence: M(n+1) = 2*M(n) + 1
4. Cullen numbers, https://en.wikipedia.org/wiki/Cullen_number
General form: C(n) = n*2^n + 1
Recurrence: C(n+1) = 4*C(n) + 4*C(n-1) + 1
5. Hampshire numbers: (My own choice of name)
General form: H(n,b) = (n+1)*b^n - 1
Recurrence: H(n+1,b) = 2*b*H(n-1,b) - b^2*H(n-2,b) - (b-1)^2, H(0,b) = 0, H(1,b) = 2*b-1
6. Tin numbers, so named because Sn is the atomic symbol for tin.
General form: S(n) = 2*n*F(n) + 1, where F(n) is the nth Fibonacci number.
Recurrence: S(n) = S(n-5) + S(n-4) - 3*S(n-3) - S(n-2) +3*S(n-1);
To wrap thing up, I hope you have enjoyed this beginning of a journey into large primes and non-primes. I've shown a few ways we can use roughness, first in a constructive way to identify numbers which may harbor primes in a greater density than would otherwise be expected. Next, using GCD in a very pretty way, and finally by use of MOD and the full power of MATLAB to test elements of a sequence of numbers for potential primality.
My next post will delve into the world of Fermat and his little theorem, showing how it can be used as a stronger test for primality (though not perfect.)